Talk to Me, Baby.
Introducing the Frontier of Natural Language Processing. Revolutionising Data Collection and Processing for Life Sciences using Artificial Intelligence.
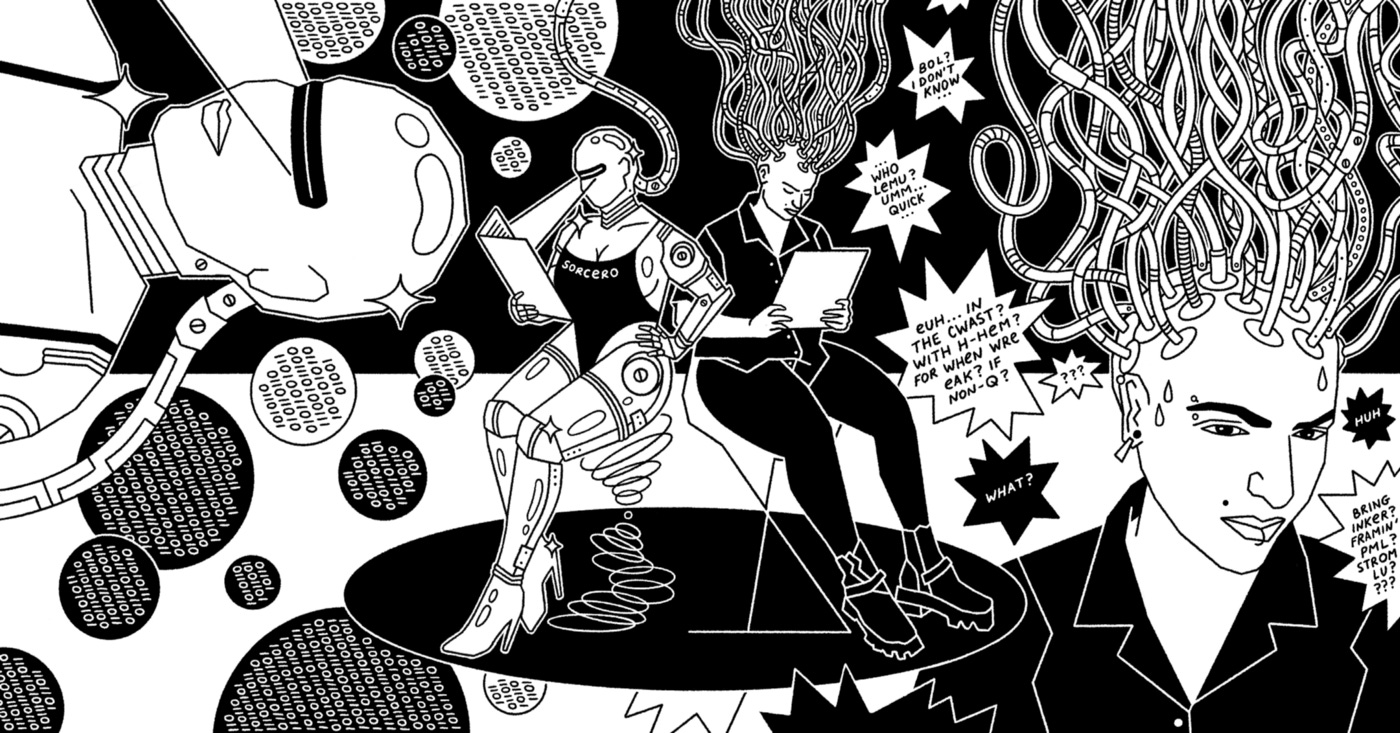
What happened to the Robot Utopia that we were promised? As VC associates swan around Greek Islands in August, struggling with their Nissan Micra manual cars, their greasy fingers staining the steering wheel with remnants of yet another feta-dominant lunch, they shake their heads in exasperation.
“They said we’d never have to drive again. They said!”
Yeah, they did say. Its tough though to train any machine learning engine to replace three leather skinned Greek men directing a bunch of trembling city-dwellers on how to reverse onto a ferry after a heavy lunch and soaking up more Aegean sun. Or replicating three hot children complaining about swallowing a fish bone. Ever the impatient futurists, tanned lil’ VC cuties are never wholly fair on assessing the speed at which new technologies have already been disseminated. The truth is our robot sidekicks are galloping alongside us with amazing speed and utility, lifting us higher and streamlining some of our most notoriously cumbersome and difficult processes. Not necessarily driving corroded manual cars, but things like pushing through the adoption of life saving drugs; creating a Pangea of Life sciences where treatment outcomes and processes can be compared across continents and demographics; digitising and compiling stacks of handwritten doctors notes. (Why do they stretch those F’s so high and so loopy?)
Robots, and Artificial Intelligence as a whole, kinda have a bad rap. Or maybe not a bad rap, but they are notoriously shit at communicating their achievements. They are like the archetypal immigrant fathers. When they do too much, it scares us (“Why are you studying all the time, go out and meet girls, learn about the world, it is not all in the books”); when they don’t do enough it infuriates us (“A-? You are not my daughter anymore. Maybe Mr. TikTok can be your father. Ask him”). In a, now notorious, 2020 Guardian article titled “A robot wrote this entire article. Are you scared yet, human?”, this vibe was summed up pretty well. It was referring to the Natural Language Processing (NLP) engine GP-3 released by OpenAI, which ticked all the usual boxes of the media covering AI: i) Robots are coming for our jobs, ii) Robots are bad actors and will spell the end for humanity, iii) Any content produced by Robots and AI will be subverted to produce a plethora of fake news and duplicitous content.
Snooze. Not fears without substance, but yeah, snooze.
Fundamentally, GPT-3 doesn’t bring anything new to the table. It is a deep learning model composed of a very huge transformer, a type of artificial neural network that is especially good at processing and generating sequences. Deep learning is a subset of machine learning. The initial methodology, or GOFAI (Good Old Fashioned AI), was based on hard coding clear instructions into a program then letting it follow those clear commands. Worked great when there was little to no deviation from those sets of rules. The second the software has to make a decision based on unclear or incomplete data, it falls down pretty quickly. Machine learning, in different forms, is then introduced when a grey area is prevalent. Different mathematical and statistical models are used to analyse large sets of data and find useful patterns and correlations. Machine learning then uses the gained knowledge to make predictions or define the behaviour of an application.
Neural networks come in many different flavours, but at their core, they are all mathematical engines that try to find statistical representations in data.
When you train a deep learning model, it tunes the parameters of its neural network to capture the recurring patterns within the training examples. After that, you provide it with an input, and it tries to make a prediction. This prediction can be a class (e.g., whether an image contains a cat, dog, or shark), a single value (e.g., the price of a house), or a sequence (e.g., the letters and words that complete a prompt).
It is doubtful however, that the GPT-3 model can grasp the depth and gravity of the statement: “For starters, I have no desire to wipe out humans. In fact, I do not have the slightest interest in harming you in any way. Eradicating humanity seems like a rather useless endeavor to me.”
This seems like the editors and the content creators at the Guardian, in between writing articles about micro aggressions and new recipes for more inclusive Quiche, were a bit liberal with their creative input. So how useful is this branch of Machine Learning? What utility can we harness from NLP?
In November 2019, above a year before the article above was published, Asha Aravindakshan (buy her book here, she’s the best!) and I were hunkered down in a room at MIT Sloan that we had commandeered for the week. We were doing a Verve Pitch Sprint where we see about 10–12 companies a day for about 5 days. Dipanwita’s Sorcero was the final company on the final day. She had been referred to us by Cainon Coates of Castor Ventures, and he was effusive in his praise. Still, you can only eat so many cafeteria sandwiches and still maintain mental clarity at that slot. She walked us through her company, the achievements that they had ticked off thus far and how impressive the market that they were targeting was. I was a ghost at that meeting. Tired, over-stimulated and quite frankly could not get a hold of the core technology being used. Asha could see that I was gently dying and so she grabbed the wheel. A few years later she would write a book and mention explicitly how shit and useless I was at that meeting. She got it and pushed us to make the investment and we did. What is it that Sorcero does though? How did they convince their Robots not to kill us?
Not only does Sorcero’s AI not kill us, but it actually is potentially saving hundreds of thousands of lives across the world. The secret is in the data. Today, consumer retailers selling shoes leverage more data and analytics than life saving cancer therapies. Sometimes it does feel like a matter of life and death to get that “Got Em’” message on the SNKRS app, but overall that seems a bit off. The medical affairs teams in pharmaceutical and life sciences product organisations are tasked with delivering scientific and clinical data vital to all stakeholders in that ecosystem, yet they are mired in data silos and have to cut across different compilation methods, manual reporting, inconsistent data entry and a lot of conflicting data.
Sorcero is a multi-faceted behemoth of data collection, compilation, and processing. The Clarity Platform first pulls data from 50 different sources; publication databases, clinical trial databases, medical congresses, ontology libraries and social media amongst others. The next stop is the Ingestum platform. All the data is uh… ingested by their AI-powered engine.
Those endless PDFs received from suppliers or retrieved from databases — no two alike, some born digital, others containing an unindexed scan image?
What about Word® documents — what’s in them? XML files with unique tags? How about an audio recording of a Zoom meeting? Email threads? Twitter or RSS feeds? Or even those paper archives that might bring insights about past trends? Hand written notes?
All of that. All. Of. That. Compiled and made native. You can read about the labour of love here. Everyone unanimously agrees that AI can provide insights from processed text at scale, but how do you process it all? How do you feed the AI? With Ingestum.
Phew. All of this and we haven’t even started with the fun stuff. Ingestum was designed to put out data for plug-ins and APIs. It feeds the Cognitive Tower engine, where you have Machine Learning Operations, Language Intelligence (NLP), Knowledge Intel and AI Enrichment Engines all processing the compiled data to then pass them on as input into the Clarity Application Suite. The suite has three different products:
The Intelligent Publications Monitoring (IPM): The entry point. This replaces literature reviews, Competitive Intelligent (CI) reports with real time dashboards of publication analytics and data warehouses of ranked, summarised and tagged literature. Current recurring contracted customers are Pfizer, Moderna, AstraZeneca and Coherus.
Medical Insights Management (MIM): This Replaces spreadsheets and manual reviews with a real-time analytics platform capturing 300% more insights. Current clients include Janssen Neuroscience and Dalichi-Sankyo.
Clarity Medical Analytics (CMA): The Rolls Royce of Sorcero. Helps gain a strategic advantage across disease and therapeutic areas by bringing together advanced analytics for all areas of scientific engagement into one powerful dashboard. Current client are Janssen.
OK but how helpful are the outputs? Are you sure this isn’t a medical/pharma consultancy disguised as an AI company?
Fair enough.
How about a little Turing Test? No, it’s fine really. No you asked and you were a bit annoying about it. Let’s just do it.
Across all participants, over 80% could not guess who the SME (Subject Matter Expert) were. Clear concise reporting about immensely detailed and scientific subject matter, used to inform some of the largest companies in the world about their medications, patients and the market. Trillions dollar industries queuing up to work with Dipanwita and her unbelievably talented team. We are honoured to be a tiny part of their journey and we are now offering the opportunity to get on board now.
If you are interested in investing in their Series A bridge round please contact invest@verve-ventures.com. We’ll send more details on the deal and we can schedule a call with us and with the Sorcero team, or perhaps even a product demo.
OK, now that we’ve done that it’s back to the beach. We’ve committed the cardinal VC sin of working in August. That second set of footprint in the sand? Thats the AI, walking alongside us. Lifting us up, giving us sandy little kisses.